At OpenAsset, we’re constantly striving to maintain our position as the No.1 Digital Asset Management provider for companies operating in the Architecture, Engineering and Construction (AEC), and Real Estate industries. Our platform is designed to help companies passionate about winning business by enabling any member of an organization to access their best image assets. One of the keys to our success has been our ability to continually develop and improve our product in ways that anticipate the needs of our 60,000 users.
Artificial Intelligence (AI) is already reshaping the way businesses operate across many industries. By automating processes that previously required human input, companies stand to make significant savings in time and money. However, AI remains an emerging technology and its strengths and weaknesses require focussed assessment in order to provide value. In this instalment in a series of articles on AI, we will explore the period of research and development that helped us to identify the key applications of AI that would be most valuable to our clients.
Product Roadmap
The product team at OpenAsset are continuously working on a range of projects that are at different stages of research and development. The focus of our work is to establish the problems that customers face and through stages of exploration, arrive at solutions that drive customer progress.
The image below shows our Product Roadmap, which features all projects currently underway. The board is divided between themes that are in a discovery (research) or delivery phase, moving from left to right as they develop from ideas to workable features that are ready to launch.
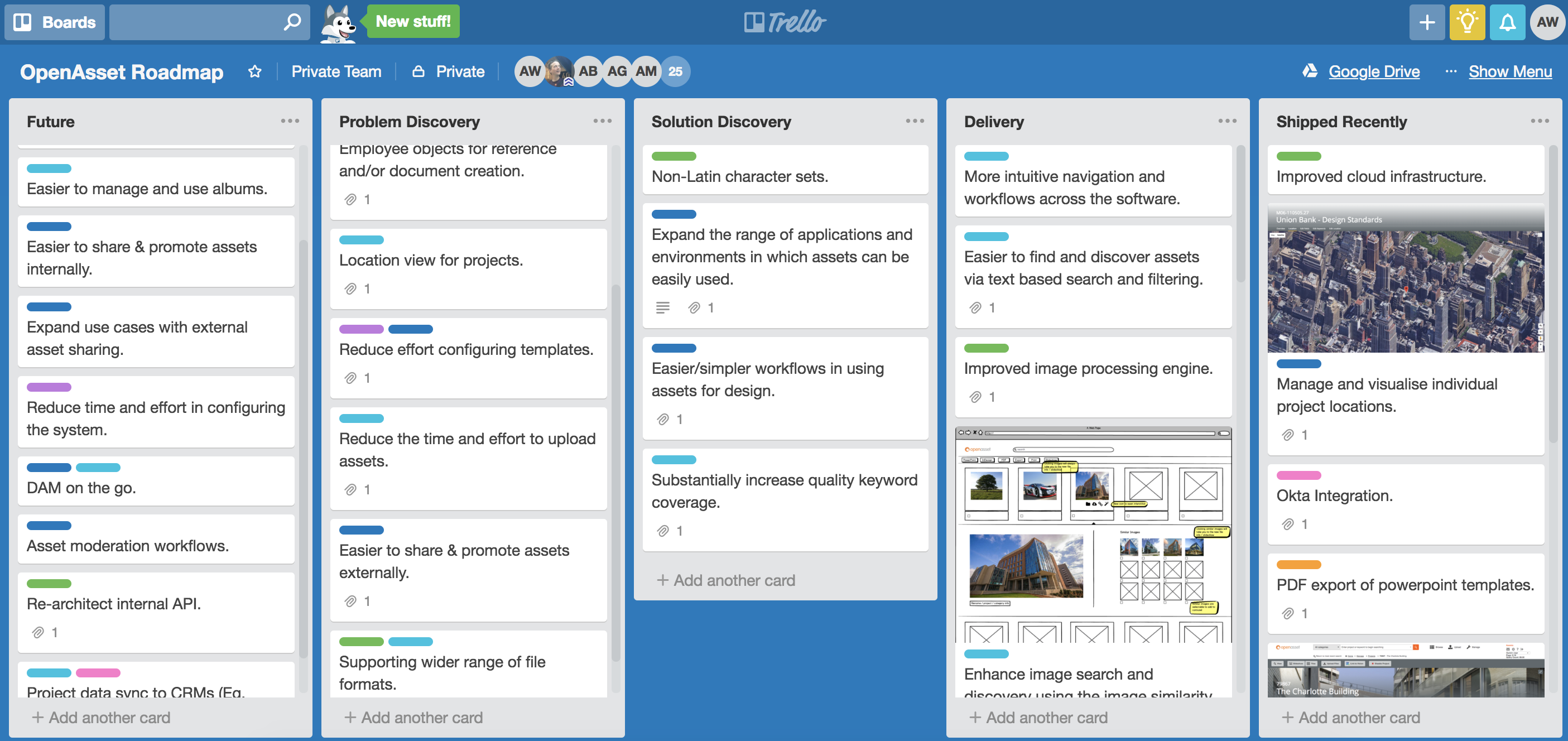
In order to establish how Artificial Intelligence and image recognition could be harnessed to help our customers, we have tested and experimented with the technology over a period of 18 months and we are committed to continue this into the future.
We focussed our early investigation on two key questions:
- Can we save our customers time and money by assisting or automating the tagging of images?
- Are there opportunities with this emerging technology to assist our customers in finding images?
Our research has benefitted from an approach focussed on the needs of our customers within the AEC and Real Estate industry. Working in small teams, we have been able to make decisions quickly and react to new discoveries. This has led us to adopt AI technology in a way that we believe differs from that of other DAM vendors.
Comparing Image Recognition Platforms
Having established some key objectives, our next step was to compare the image recognition platforms that have been launched recently by key industry players such as Amazon Web Services (Rekognition), Google Cloud (Vision) and Clarifai. As an established SaaS provider, we have a solid understanding of how people use our product and we tested the different vendors using assets that our customers typically store. These include images of building exteriors, interiors, details and plans. We concluded that while there are differences between results from each platform, there is no discernible performance advantage when analysing images from the built environment.
The Shortcomings of AI Services
Most image recognition services are based on models trained to recognise objects against a wide array of imagery, often leading to generic keyword suggestions when industry-specific images are analysed. As customers are likely to be searching for specific features of an image, these generic terms are unlikely to improve the quality of their search.
For example, while Amazon Rekognition can easily identify that an image features a building or a bridge, it cannot reliably distinguish between a school and a hospital, or between a corridor and an atrium. It is precisely this contextual information that is of value to our clients.
Another shortcoming of image recognition is the inability to distinguish between the pertinent and non-pertinent information within an image. The presence of an airplane in the skyline of an image might lead to the incorrect suggestion that the building is an airport. The inaccuracy of such suggestions could lead to errors in image tagging, or would necessitate manual input to select only relevant keyword suggestions.
Data Analysis
By comparing the keyword suggestions of the image recognition platforms against those typically used by our customers, we concluded that the suggestions from Amazon Rekognition were too generic and lacked the specificity required to bring value to AEC or Real Estate users. While some keyword suggestions were correct, many were too ambiguous to be useful and often suggestions were incorrect.
Moving onto the next phase of testing, we believed that the image recognition keyword suggestions would be useful in two key respects:
- The suggestions could be used to calculate the visual similarity between images
- Through developing an AEC and Real Estate-specific ontology, keyword suggestions could be improved into useful search terms
In the following article, we will explore how these insights helped us to move forward with our product development.